Ever wonder how your phone knows what you want to write next? Or how Netflix recommends shows you might enjoy? This is all made possible by artificial intelligence, or AI. AI is transforming the way we live and work, from healthcare to entertainment. Its becoming more and more of a deal every day! Yet who are the brains behind these intelligent systems? The answer is a bit like a movie with a whole lot of different actors! Yes, the building and running of AI requires a diverse AI cast.
The Data Architects: Building the Fuel for the AI Engine
AI consumes food in the form of data! Without it, AI systems learned or made decisions. Consider data architects the farmers. They cultivate and collect the raw materials required to feed these AI brains. These individuals are critical for ensuring that the data that is collected is of high quality so that it can be effectively managed and made ready for use. So, data architects prepare, gather, and store data in AI systems.
Data Acquisition: Where the Raw Material is Collected
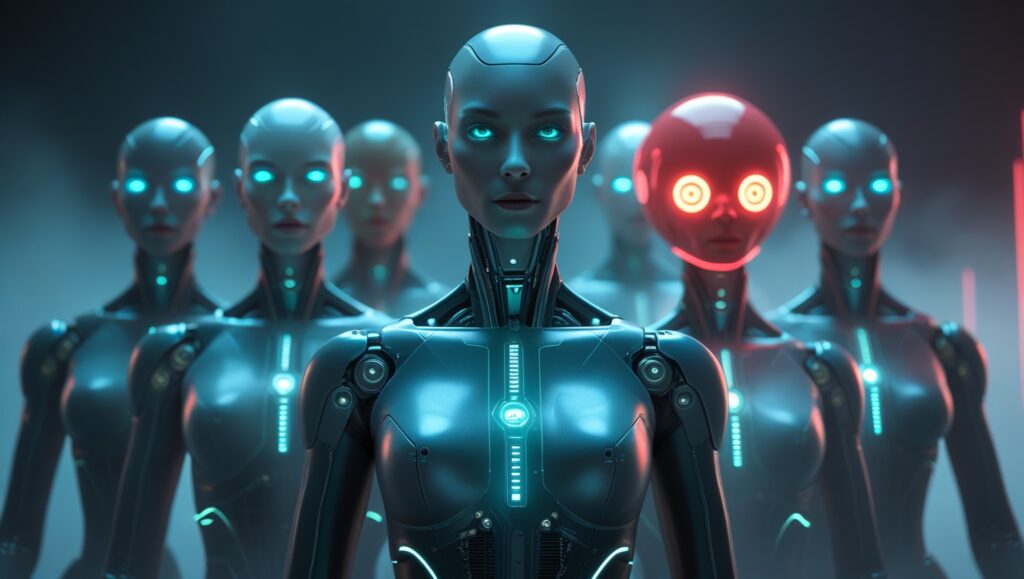
Data Acquisition — It’s all about gathering the data! There are multiple ways of going through this process. One is web scraping, meaning that data is scraped from websites. Another one is sensor data, where devices collect information from the real world. This is also generated using user content. These can be posts in social media But, it’s not always easy. We must consider ethics Overlap with other things that impact treatment. Privacy is super important. All data gathering needs be careful that they are not invading people’s privacy.
Data Wrangling: Cleaning and Preparing the Data
So, we have all this data, now what do we do with it? That’s where data wrangling comes in.Data wrangling is like organizing a messy room. Data cleaning, transformation, and merging are involved. That helps make it valuable for AI models. And if data is a mess, then AI is not going to work. This is why data wrangling is significant. It keeps data consistent.
ETL (Extract, Transform, Load) Process: Preparing Data for Storage
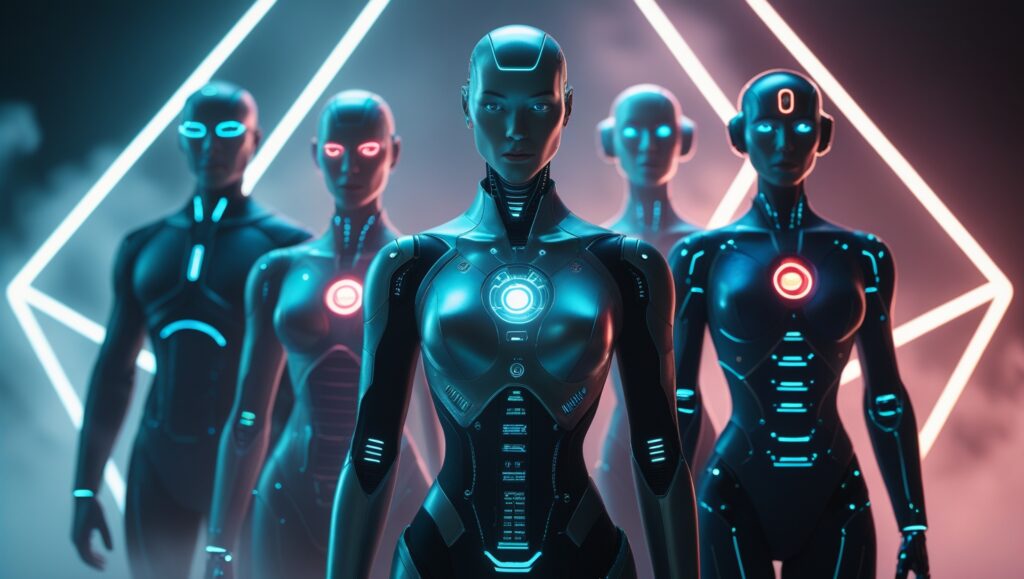
Pretend there’s a really big warehouse with all the data in the world that AI would ever need. That is a data storage and management! It means safely storing it. It’s about keeping it organized. The data is stored in solutions known as data lakes and data warehouses. Data governance is extremely important. It means setting the rules for how data is employed. Data security is also key. And, we have to keep data safe from theft.
ROLE 2: The Algorithm Engineers: Enabling the algorithm logic
Algorithm engineers are the experts behind the AI. They design and develop the models that bring AI to life. These folks are coders and problem-solvers. Their work is very important. They are key to how successful an A.I. will be.
Predictive Modeling: Training Machine Learning Models
The AI learns with the help of machine learning. Now, there are different types of machine learning algorithms. Supervised learning requires the model to be trained on labeled data. Unsupervised learning discovers structures in unlabelled data. Reinforcement learning models are trained through trial and error. The model gets trained by the engineer. Then, they evaluate it. Finally, they deploy it.
Neural Network Designs: Imitating the Human Brain
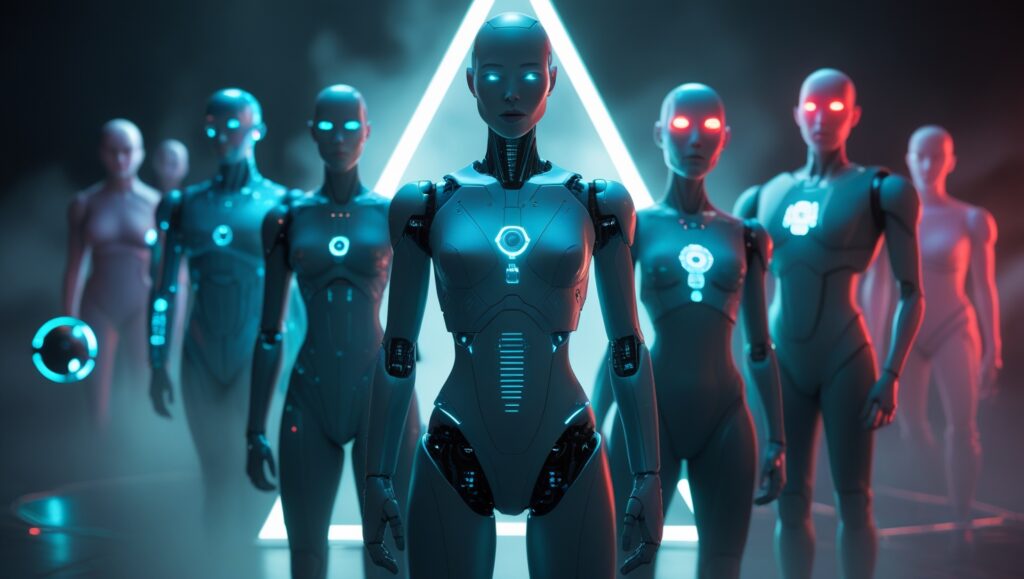
Animal Learning with Specific Subject: Deep Learning It uses neural networks. The neural-net is a bit like the human brain. Convolutional neural networks (CNNs) excel at image recognition. The Recurrent neural networks (RNNs) is very suitable for the natural language. Many applications of artificial intelligence rely on deep learning. Ranging from image recognition to language processing.
Natural Language Processing (NLP): Teaching AI to Understand Language
NLP enables AI to comprehend and analyze language. It’s how your phone understands what you’re saying. Sentiment analysis determines whether a piece of text is positive or negative. The first one is general text summarization that creates short forms of long articles. Machine learning translates languages. AI becomes a good communicator with the help of NLP.
Infrastructure Providers: The Driving Force Behind the AI Revolution
You can have data and you can have AI but without the proper tools to handle them neither will work. Hardware and software are among these tools. Infrastructure providers build. They offer these critical resources. Without it, AI could do very little.
Cloud-based Platforms for AI: Unlocking Scalability and Flexibility
Cloud Computing resources are made available to computers and other devices on demand via the Internet. Amazon, Microsoft, and Google are major providers of AWS, Azure, and GCP respectively. They provide services that are specific to AI. Cloud computing is beneficial for AI. It offers scalability. It is also cost-effective. These types of platforms give you access to cutting-edge hardware.
Availability of specialized hardware to accelerate AI.
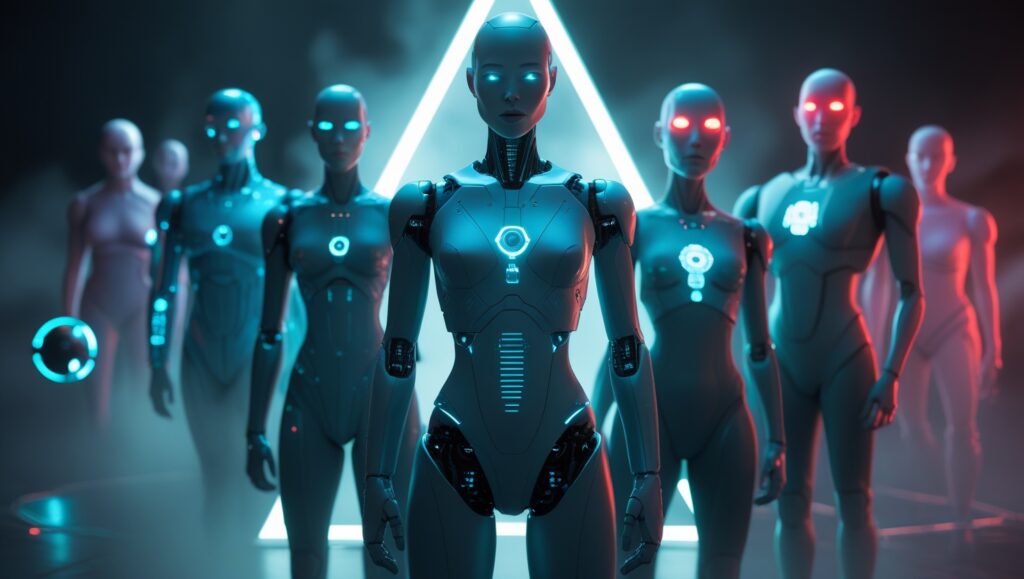
AI can be resource intensive. Its performance requires specialized hardware. GPUs and TPUs are common. They speed up AI tasks. They train and infer on specialized hardware. The outcome? A quicker, more efficient AI.
The Guardians of Ethics: Making sure AI is not Misused
AI can be powerful and AI definitely has its risks. You had to think about the ethics of it all. Ethics guardians answer those questions. That encompasses things like fairness, bias, and transparency.
Bias Detection and Mitigation: Best Practices and Tools
AI models can be biased. It means they make biased decisions. Bias might stem from biased data. Finding and reducing bias can be approached in several ways. It is essential to promote balancing. We need accountability in AI.
The Black Box Revealed: A New Era of Explainable AI (XAI)
Sometimes AI is a black box, in a way.” We don’t understand why it makes some decisions. Explainable AI — XAI for short — seeks to address this. XAI increases the transparency of AI models. Such as importance analysis of features. These give us clues into how AI works.
Data privacy and security: safeguarding sensitive information
AI uses a lot of data. Some of it is sensitive. Securing this data is crucial. There are ways to safeguard sensitive information. Anonymization protects people in data. Encryption renders data unreadable to its unauthorized users. Access control restricts the visibility of data to whom.
Conclusion
It consists of different roles in an “AI cast. All of these roles support AI systems. Data architects are the ones who feed data to AI. Algorithm engineers write the AI logic. Infrastructure providers are the backbone of the AI revolution. Trained on data until October 2025. Ethics guardians responsible AI. Partnering to benefit from AI Responsible development of AI is also important. AI is becoming increasingly more relevant. It will transform our world.